6 May 2022 | By Madodomzi Mafanya
Large numbers of accurate reference samples are needed for training machine-learning (ML) algorithms to map the distribution of invasive alien plants (IAPs). Adequate samples help reduce overfitting by models, especially when working with data characterized by high dimensionality (e.g., hyperspectral remotely sensed satellite images). This phenomenon is known as the Hughes’ curse. However, acquiring adequate reference samples using traditional field data collection methods can be challenging due to time constraints, logistical limitations, and terrain inaccessibility.
A recent study by PhD student, Madodomzi Mafanya, C∙I∙B Core Team member Tsungai Zengeya (SANBI) and colleagues assessed if traditional image classification algorithms such as the maximum likelihood classifier (MLC), support vector machine (SVM) and spectral angle mapper (SAM) could be used as an alternative to generate adequate training samples that are required to map the distribution of the alien plants using the invasive pompom weed (Campuloclinium macrocephalum) in peri-urban areas in Gauteng as a case study.
Pompom weed was an ideal study species because its phenology makes it a good candidate for detection from hyperspectral imagery. These characteristics include a conspicuous purple-pink colour that has a very distinct spectral signature (compared to its background and co-occurring indigenous plants) and the plant forms large continuous stands instead of occurring as sparse individual herbs. This assessment used DESIS hyperspectral data that has high dimensionality (235 bands) and a moderate spatial resolution (30 m).
Using very fine resolution imagery with low dimensionality (e.g., drone colour infrared images) would have resulted in non-conclusive results. The study showed that the SAM, MLC and SVM classifiers had pixel-based classification accuracies of 87%, 73% and 67% for detecting the pompom weed, respectively.
In addition, an independent field verification survey for the SAM classification was conducted yielding a 92% overall mapping accuracy for detecting the pompom weed. A total of 4 000 pompom weed and 8 000 non-pompom weed training samples were generated from an SAM classification that was trained using only 20 pompom reference samples.
The findings demonstrate that it is possible to generate large numbers of accurate training samples from traditional image classification algorithms that can be used to map the distribution of alien plant species using machine-learning algorithms.
Read the full paper
Mafanya M, Tsele P, Zengeya T, Ramoelo A (2022) An assessment of image classifiers for generating machine-learning training samples for mapping the invasive Campuloclinium macrocephalum (Less.) DC (pompom weed) using DESIS hyperspectral imagery. ISPRS Journal of Photogrammetry and Remote Sensing 185: 188-200. doi:10.1016/j.isprsjprs.2022.01.015
For more information, contact Madodomzi Mafanya at u16385218@tuks.co.za
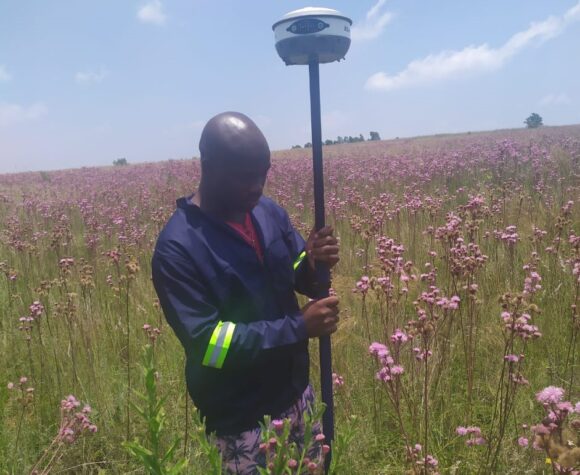